As the competition in the generative AI field intensifies, particularly with a focus on multimodal models, Meta has introduced Chameleon—a preview of its cutting-edge approach to AI. Unlike models that combine separate components for different modalities, Chameleon is designed to be natively multimodal, integrating various data types seamlessly.
Although Meta has not yet released the Chameleon models, preliminary experiments indicate that Chameleon achieves state-of-the-art performance in several tasks. These tasks include image captioning and visual question answering (VQA), where it excels, while also maintaining competitive performance in text-only tasks.
Chameleon’s innovative architecture enables a deeper understanding of both visual and textual information, unlocking new AI applications that require sophisticated interpretation across multiple data types. This advancement positions Meta at the forefront of the AI race, promising significant contributions to the development of versatile and powerful AI systems.
Early-fusion multimodal models
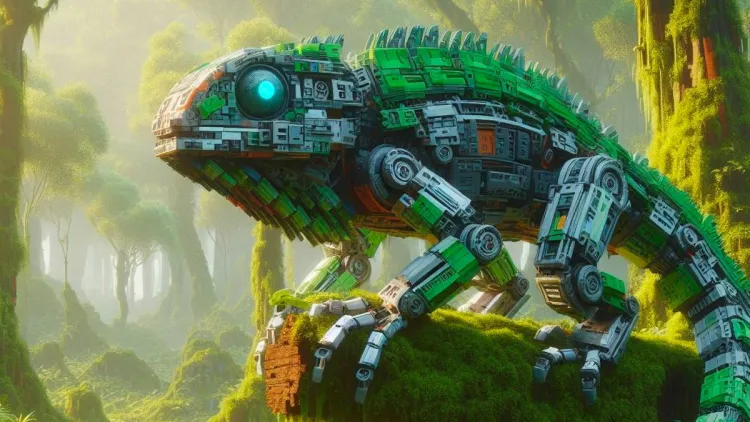
The common method for creating multimodal foundation models involves combining models that have been individually trained for different modalities, a technique known as “late fusion.” In this approach, the AI system processes various data modalities separately using distinct models and then merges their encoded representations for inference. Although late fusion can be effective, it has significant limitations. It restricts the models’ ability to integrate information seamlessly across different modalities and hinders the generation of sequences that interleave images and text. This separation can result in less cohesive and less contextually aware outputs when dealing with complex multimodal tasks.
Chameleon employs an “early-fusion token-based mixed-modal” architecture, which is specifically designed to learn from a combined mix of images, text, code, and other data types from the outset. This innovative approach transforms images into discrete tokens in a manner similar to how language models handle words. Chameleon utilizes a unified vocabulary that includes tokens for text, code, and images, enabling the application of the same transformer architecture to sequences containing both image and text tokens.
Researchers highlight that Chameleon’s most comparable counterpart is Google Gemini, which also uses an early-fusion token-based method. However, there is a key difference: while Gemini employs separate image decoders during the generation phase, Chameleon functions as an end-to-end model that processes and generates tokens without the need for distinct modality-specific components.
“Chameleon’s unified token space allows it to seamlessly reason over and generate interleaved image and text sequences, without the need for modality-specific components,” the researchers explain. This design enables Chameleon to integrate and generate multimodal content more effectively, providing a more cohesive understanding and output across different data types.
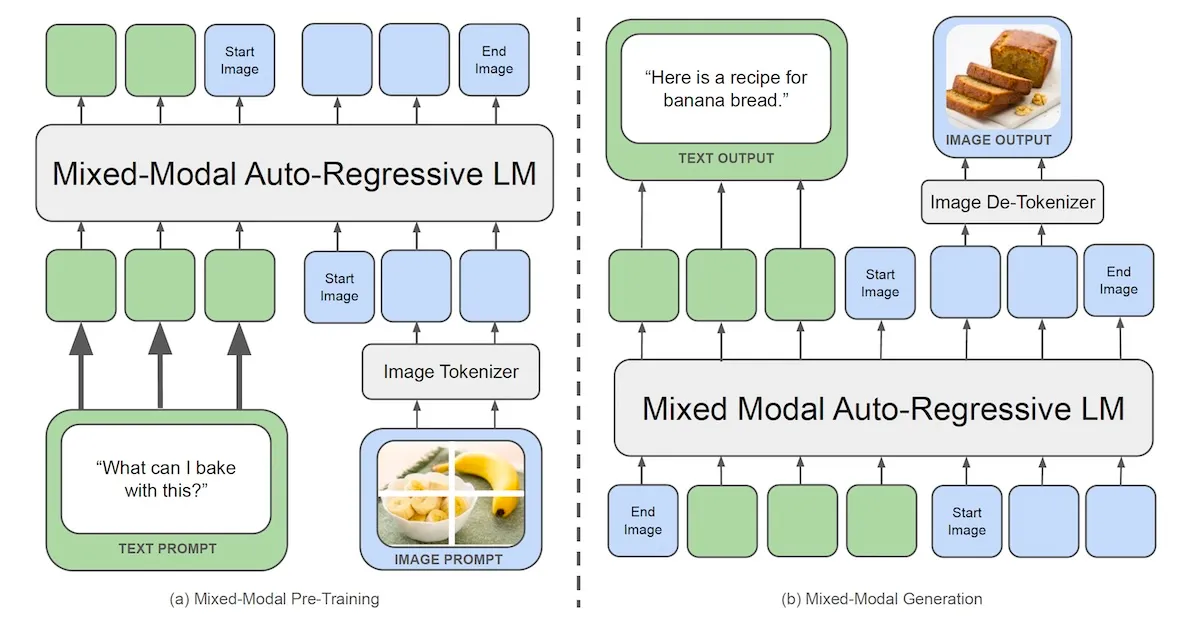
While early fusion offers substantial benefits, it also presents significant challenges in terms of training and scaling the model. To address these challenges, the researchers implemented a series of architectural modifications and advanced training techniques. In their paper, they detail various experiments and their impacts on the model’s performance.
The training of Chameleon is conducted in two stages using a dataset containing 4.4 trillion tokens, including text, image-text pairs, and interleaved sequences of text and images. The researchers trained both a 7-billion-parameter and a 34-billion-parameter version of Chameleon, utilizing over 5 million hours on Nvidia A100 80GB GPUs. This extensive training regimen ensures that Chameleon can effectively handle and integrate multiple data modalities, leading to superior performance in generating and understanding multimodal content.
Chameleon in action
According to the experiments reported in their paper, Chameleon demonstrates the capability to perform a wide array of text-only and multimodal tasks. On benchmarks for visual question answering (VQA) and image captioning, the Chameleon-34B model achieves state-of-the-art performance, surpassing models such as Flamingo, IDEFICS, and Llava. The researchers highlight that Chameleon matches the performance of other models with significantly fewer in-context training examples and smaller model sizes, both in pre-trained and fine-tuned evaluations.
One of the challenges of multimodal models is a potential performance drop in single-modality tasks. For instance, vision-language models often underperform on text-only prompts. However, Chameleon remains competitive on text-only benchmarks, matching the performance of models like Mixtral 8x7B and Gemini-Pro in commonsense reasoning and reading comprehension tasks.
Chameleon also introduces new capabilities for mixed-modal reasoning and generation, particularly for prompts requiring interleaved text and image responses. Experiments with human-evaluated responses indicate that users generally preferred the multimodal documents generated by Chameleon.
Recently, both OpenAI and Google unveiled new models offering rich multimodal experiences, but they have not disclosed much detail about their models. If Meta continues its trend of releasing model weights, Chameleon could serve as an open alternative to these private models, fostering further research and development.
Early fusion techniques like those used in Chameleon could inspire new directions in developing more advanced models, especially as additional modalities are integrated. For example, robotics startups are already exploring the integration of language models into robotics control systems. The potential for early fusion to enhance robotics foundation models is an exciting area of future research.
“Chameleon represents a significant step towards realizing the vision of unified foundation models capable of flexibly reasoning over and generating multimodal content,” the researchers write, underscoring the model’s potential to advance the field of AI significantly.
Conclusion:
Chameleon represents a significant advancement in the development of multimodal AI models, seamlessly integrating various data types and demonstrating superior performance across a range of tasks. By adopting an early-fusion architecture, Chameleon overcomes many limitations of traditional late-fusion models, unlocking new capabilities for mixed-modal reasoning and generation. With its potential for broad application and further research, Chameleon is poised to make substantial contributions to the field of AI.
If you’re seeking similar services in programming, contact Arcitech.ai. We assure you the best and most innovative solutions for your business.