In a groundbreaking moment for the field of AI Pioneers like the Royal Swedish Academy of Sciences has awarded the Nobel Prize in Physics to Geoffrey Hinton and John Hopfield for their pioneering work in machine learning. This prestigious recognition not only celebrates their individual contributions but also marks a significant milestone in acknowledging the profound impact of artificial intelligence and machine learning on modern society.
The Laureates: Visionaries in AI Pioneers
Geoffrey Hinton AI Pioneer : The “Godfather of AI”
Geoffrey Hinton, 76, widely known as the “Godfather of AI,” has been a towering figure in the field of machine learning for decades. His work on neural networks and deep learning algorithms has been foundational to many of the AI technologies we use today1. Hinton’s journey in AI began in the 1970s when the field was still in its infancy, and many were skeptical about the potential of neural networks.
Hinton’s persistence and innovative thinking led to several breakthroughs, including the development of the backpropagation algorithm, which is crucial for training deep neural networks2. His work on deep learning, particularly in the areas of image and speech recognition, has been instrumental in advancing AI capabilities to levels that were once thought impossible.
In 2023, Hinton made headlines when he resigned from his position at Google, citing concerns about the potential dangers of AI advancements3. His warning about machines potentially outsmarting humans sparked intense debate within the tech community and beyond, highlighting the ethical considerations that come with rapid AI development.
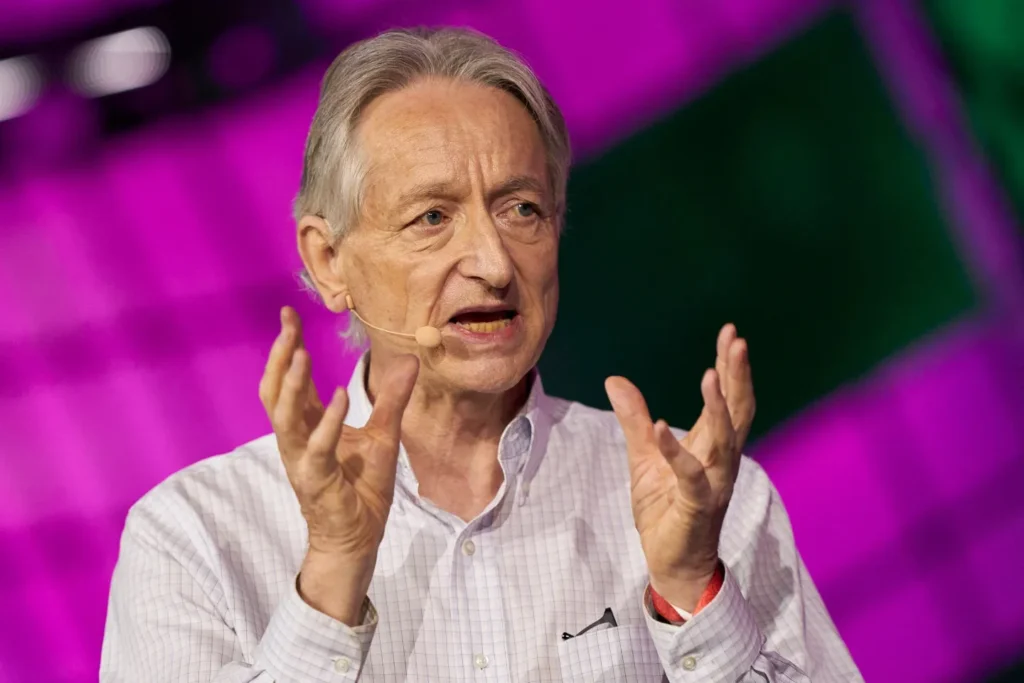
John Hopfield: The Physicist Who Bridged Disciplines
John Hopfield, 91, from Princeton University, brings a unique perspective to the field of machine learning. As a physicist, Hopfield’s work exemplifies the power of interdisciplinary research. His contributions have been crucial in understanding how neural networks can exhibit collective computational abilities, similar to those observed in biological systems4.
Hopfield is perhaps best known for his invention of the Hopfield network, a form of recurrent artificial neural network. This model, introduced in the early 1980s, demonstrated how artificial neural networks could be used to store and retrieve memory patterns, mimicking the associative memory of the human brain5.
His work has not only advanced our understanding of artificial neural networks but has also provided valuable insights into the functioning of biological neural networks. This cross-pollination of ideas between physics, neuroscience, and computer science has been a hallmark of Hopfield’s career and has significantly influenced the development of machine learning algorithms.
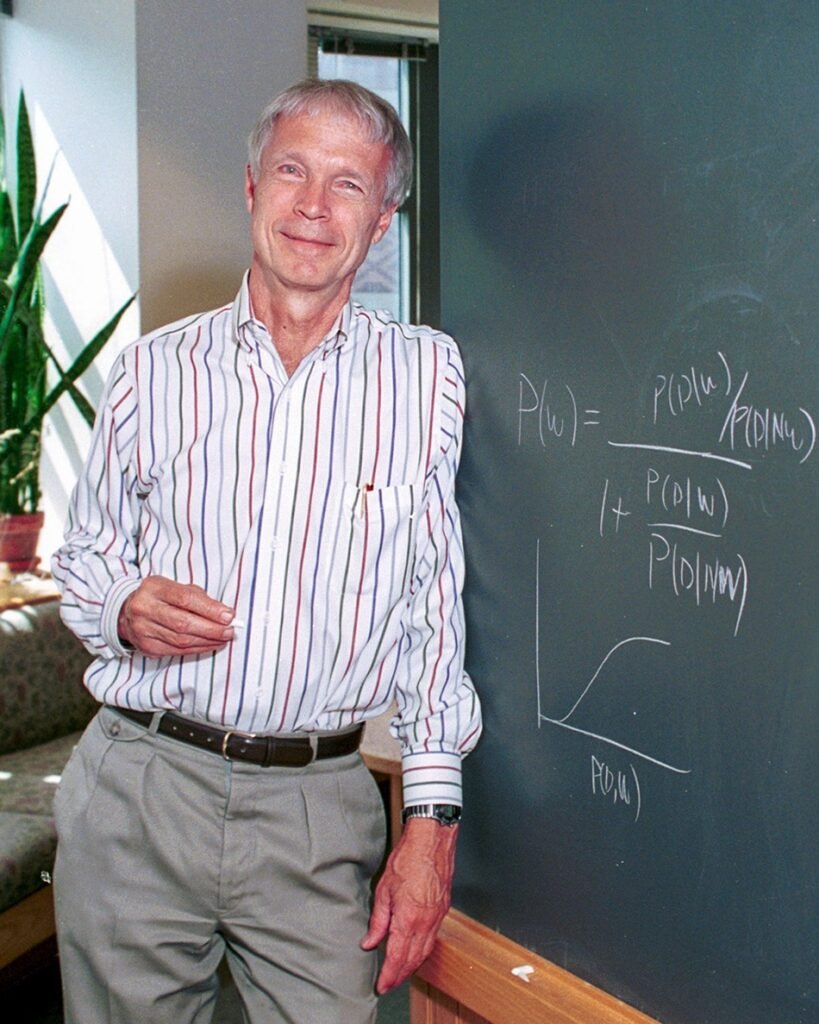
The Impact of Their Work
The contributions of Hinton and Hopfield have been instrumental in shaping the modern landscape of artificial intelligence and machine learning. Their work underpins many of the technologies we interact with daily, from voice assistants and recommendation systems to advanced medical diagnostics and autonomous vehicles.
Revolutionizing Image and Speech Recognition
One of the most visible impacts of their work is in the field of image and speech recognition. Hinton’s research on deep learning has led to dramatic improvements in these areas. Today, AI systems can recognize images and understand speech with accuracy that rivals or even surpasses human capabilities in some contexts6. This has enabled applications ranging from facial recognition systems in smartphones to advanced medical imaging analysis that can detect diseases early.
Enhancing Natural Language Processing
The principles developed by both laureates have also been crucial in advancing natural language processing (NLP). Modern AI systems can now understand and generate human-like text, translate between languages, and even engage in complex conversations. These capabilities are powering chatbots, automated content generation, and sophisticated language translation services7.
Advancing Scientific Research
Beyond consumer applications, the machine learning techniques pioneered by Hinton and Hopfield are accelerating scientific research across various fields. In drug discovery, AI models are helping to predict protein structures and identify potential new medicines8. In climate science, machine learning algorithms are improving climate models and helping to analyze vast amounts of environmental data9.
Transforming Industries
The impact of machine learning extends to nearly every industry. In finance, AI algorithms are used for fraud detection and algorithmic trading. In manufacturing, machine learning enables predictive maintenance and quality control. The automotive industry is leveraging these technologies for the development of self-driving cars10. The list of applications continues to grow, demonstrating the far-reaching influence of the laureates’ work.
The Nobel Prize: A Recognition of AI’s Importance
The decision to award the Nobel Prize in Physics to researchers in machine learning signifies a pivotal moment in the scientific community’s recognition of AI’s importance. Traditionally, the Physics prize has been awarded for discoveries in areas such as particle physics, quantum mechanics, or cosmology. By honoring Hinton and Hopfield, the Nobel Committee acknowledges the profound impact that artificial intelligence and machine learning are having on our understanding of complex systems and information processing.
This recognition also highlights the interdisciplinary nature of modern scientific research. Machine learning, while rooted in computer science and mathematics, draws heavily from principles of physics, neuroscience, and cognitive science. The work of Hinton and Hopfield exemplifies how insights from diverse fields can converge to create powerful new paradigms for understanding and manipulating information.
Challenges and Ethical Considerations
While celebrating the achievements of Hinton and Hopfield, it’s crucial to address the challenges and ethical considerations that come with the rapid advancement of AI technologies. Hinton’s decision to leave Google and speak out about the potential dangers of AI underscores the complex relationship between scientific progress and societal impact.
The Double-Edged Sword of AI Advancement
As AI systems become more sophisticated, questions arise about their potential to surpass human intelligence in various domains. This prospect, often referred to as artificial general intelligence (AGI), brings both excitement and concern. On one hand, AGI could lead to unprecedented scientific breakthroughs and solutions to global challenges. On the other, it raises questions about control, accountability, and the potential displacement of human workers11.
Privacy and Data Concerns
The success of modern machine learning algorithms often relies on vast amounts of data. This has led to growing concerns about privacy and data protection. As AI systems become more integrated into our daily lives, there’s an increasing need for robust frameworks to ensure that personal data is used ethically and with proper consent12.
Bias and Fairness in AI Systems
Another critical issue is the potential for bias in AI systems. Machine learning algorithms can inadvertently perpetuate or amplify existing societal biases if they are trained on biased data sets. Ensuring fairness and equity in AI applications, particularly in sensitive areas like hiring, lending, and criminal justice, remains a significant challenge for researchers and policymakers13.
The Need for Responsible AI Development
The work of Hinton, Hopfield, and their colleagues has opened up tremendous possibilities, but it also calls for responsible development and deployment of AI technologies. This includes:
- Transparent and explainable AI systems
- Robust safety measures and testing protocols
- Ethical guidelines for AI research and application
- Interdisciplinary collaboration to address societal impacts
- Ongoing public dialogue about the role of AI in society
Looking to the Future
As we celebrate the achievements of Hinton and Hopfield, we also look to the future of machine learning and artificial intelligence. Their groundbreaking work has laid the foundation for continued innovation in the field.
Emerging Trends in AI Research
Several exciting areas of research are building upon the foundations laid by Hinton, Hopfield, and their contemporaries:
- Quantum Machine Learning: Researchers are exploring how quantum computing principles can be applied to machine learning, potentially leading to exponential speedups in certain types of computations14.
- Neuromorphic Computing: Inspired by the structure and function of biological neural networks, neuromorphic computing aims to create more efficient and adaptable AI systems15.
- Federated Learning: This approach allows for machine learning models to be trained across multiple decentralized devices or servers holding local data samples, addressing privacy concerns and enabling more collaborative AI development16.
- Explainable AI (XAI): As AI systems become more complex, there’s a growing focus on developing methods to make their decision-making processes more transparent and interpretable17.
- AI for Scientific Discovery: Machine learning is increasingly being used to accelerate scientific research, from particle physics to materials science, opening up new avenues for discovery18.
The Role of AI in Addressing Global Challenges
The potential of machine learning to address pressing global issues is immense. Some areas where AI is expected to play a crucial role include:
- Climate Change Mitigation: AI can optimize energy grids, improve climate models, and accelerate the development of clean energy technologies19.
- Healthcare and Medicine: From drug discovery to personalized treatment plans, AI has the potential to revolutionize healthcare delivery and medical research20.
- Education: Adaptive learning systems powered by AI could provide personalized education at scale, addressing educational inequalities21.
- Sustainable Agriculture: AI can help optimize crop yields, reduce waste, and develop more resilient farming practices22.
- Disaster Response and Management: Machine learning models can improve early warning systems and coordinate more effective disaster response efforts23.
Conclusion: A New Era of Scientific Discovery
The awarding of the Nobel Prize in Physics to Geoffrey Hinton and John Hopfield marks a watershed moment in the history of artificial intelligence and machine learning. It recognizes not only their individual contributions but also the field’s immense impact on science, technology, and society at large.
As we stand on the brink of new frontiers in AI research, the work of these pioneers reminds us of the power of human ingenuity and the importance of collaborative, interdisciplinary research. Their achievements have opened up possibilities that were once the realm of science fiction, from machines that can see and understand language to systems that can learn and adapt in ways that mimic biological intelligence.
However, with great power comes great responsibility. The rapid advancement of AI technologies brings with it a host of ethical, social, and philosophical questions that we must grapple with as a society. How do we ensure that AI is developed and deployed in ways that benefit humanity as a whole? How do we address issues of bias, privacy, and the potential economic disruptions that may come with increased automation?
The recognition of Hinton and Hopfield’s work by the Nobel Committee serves as a call to action for the scientific community, policymakers, and society at large. It underscores the need for continued investment in AI research, coupled with thoughtful consideration of its implications. As we celebrate this milestone, we must also commit to shaping a future where the transformative power of artificial intelligence is harnessed for the greater good.
In the end, the story of machine learning is a testament to human curiosity, creativity, and perseverance. From the early skepticism faced by neural network research to today’s AI-driven world, the journey has been one of continuous innovation and discovery. As we look to the future, the work of Hinton, Hopfield, and countless others in the field promises to unlock new realms of knowledge and capability, forever changing our understanding of intelligence, computation, and the very nature of problem-solving.
The Nobel Prize awarded to these visionaries is not just a recognition of past achievements, but a beacon lighting the way toward a future where the boundaries between human and artificial intelligence continue to blur, opening up unprecedented possibilities for scientific discovery and human progress.
References
Footnotes
- LeCun, Y., Bengio, Y., & Hinton, G. (2015). Deep learning. Nature, 521(7553), 436-444. ↩
- Rumelhart, D. E., Hinton, G. E., & Williams, R. J. (1986). Learning representations by back-propagating errors. Nature, 323(6088), 533-536. ↩
- Metz, C. (2023). A.I. Pioneer Quits Google and Warns of Danger Ahead. The New York Times. ↩
- Hopfield, J. J. (1982). Neural networks and physical systems with emergent collective computational abilities. Proceedings of the National Academy of Sciences, 79(8), 2554-2558. ↩
- Hopfield, J. J. (1984). Neurons with graded response have collective computational properties like those of two-state neurons. Proceedings of the National Academy of Sciences, 81(10), 3088-3092. ↩
- He, K., Zhang, X., Ren, S., & Sun, J. (2016). Deep residual learning for image recognition. In Proceedings of the IEEE conference on computer vision and pattern recognition (pp. 770-778). ↩
- Devlin, J., Chang, M. W., Lee, K., & Toutanova, K. (2018). Bert: Pre-training of deep bidirectional transformers for language understanding. arXiv preprint arXiv:1810.04805. ↩
- Jumper, J., Evans, R., Pritzel, A., et al. (2021). Highly accurate protein structure prediction with AlphaFold. Nature, 596(7873), 583-589. ↩
- Rolnick, D., Donti, P. L., Kaack, L. H., et al. (2022). Tackling climate change with machine learning. ACM Computing Surveys, 55(2), 1-96. ↩
- Grigorescu, S., Trasnea, B., Cocias, T., & Macesanu, G. (2020). A survey of deep learning techniques for autonomous driving. Journal of Field Robotics, 37(3), 362-386. ↩
- Bostrom, N. (2014). Superintelligence: Paths, dangers, strategies. Oxford University Press. ↩
- Zuboff, S. (2019). The age of surveillance capitalism: The fight for a human future at the new frontier of power. Profile books. ↩
- Barocas, S., & Selbst, A. D. (2016). Big data’s disparate impact. California Law Review, 104, 671. ↩
- Biamonte, J., Wittek, P., Pancotti, N., et al. (2017). Quantum machine learning. Nature, 549(7671), 195-202. ↩
- Schuman, C. D., Potok, T. E., Patton, R. M., et al. (2017). A survey of neuromorphic computing and neural networks in hardware. arXiv preprint arXiv:1705.06963. ↩
- McMahan, B., Moore, E., Ramage, D., et al. (2017). Communication-efficient learning of deep networks from decentralized data. In Artificial intelligence and statistics (pp. 1273-1282). PMLR. ↩
- Gunning, D., & Aha, D. W. (2019). DARPA’s explainable artificial intelligence program. AI Magazine, 40(2), 44-58. ↩
- Butler, K. T., Davies, D. W., Cartwright, H., et al. (2018). Machine learning for molecular and materials science. Nature, 559(7715), 547-555. ↩
- Rolnick, D., Donti, P. L., Kaack, L. H., et al. (2022). Tackling climate change with machine learning. ACM Computing Surveys, 55(2), 1-96. ↩
- Topol, E. J. (2019). High-performance medicine: the convergence of human and artificial intelligence. Nature medicine, 25(1), 44-56. ↩
- Holmes, W., Bialik, M., & Fadel, C. (2019). Artificial intelligence in education. Center for Curriculum Redesign. ↩
- Liakos, K. G., Busato, P., Moshou, D., et al. (2018). Machine learning in agriculture: A review. Sensors, 18(8), 2674. ↩
- Akter, S., & Wamba, S. F. (2019). Big data and disaster management: a systematic review and agenda for future research. ↩
More tech news at arcitech.ai. Stay tuned!