In today’s digital age, artificial intelligence (AI) has emerged as a transformative force, redefining how businesses operate, innovate, and compete. From simple chatbots to sophisticated data analytics tools, AI integration into applications is becoming increasingly essential for organizations seeking to streamline operations, enhance customer experiences, and gain a competitive edge. This article explores the fundamentals of AI integration, its benefits, and challenges, and a real-world case study to illustrate how AI can revolutionize application development and business growth.
1. What is AI Integration?
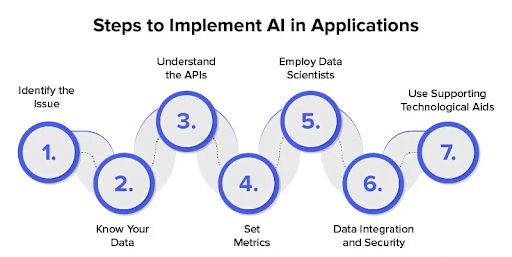
AI integration refers to embedding artificial intelligence (AI) capabilities into software applications to enhance their functionality, adaptability, and usability. This integration involves adding features like natural language processing (NLP), computer vision, predictive analytics, and machine learning to help applications become more intelligent and capable of handling complex tasks.
Here’s a closer look at some key components that are commonly incorporated in AI-integrated applications:
- Natural Language Processing (NLP): NLP enables applications to understand, interpret, and generate human language. It allows applications to comprehend voice commands, process written text, and facilitate human-like interactions. NLP powers chatbots, sentiment analysis tools, and translation services.
- Computer Vision: Computer vision allows applications to understand and interpret visual information. It’s used in image and video recognition systems, object detection, facial recognition, and quality control in manufacturing. Applications with this capability can identify patterns, detect objects, and track movements.
- Predictive Analytics: Predictive analytics utilizes historical data and statistical algorithms to identify trends and forecast future outcomes. It’s widely used for demand forecasting, fraud detection, and customer churn prediction.
- Machine Learning: Machine learning enables applications to analyze large datasets, identify patterns, and improve performance over time without explicit programming. It’s the backbone of recommendation engines, personalized marketing campaigns, and dynamic pricing models.
- Reinforcement Learning: This advanced machine learning subset involves training models to make sequences of decisions by receiving feedback on actions. It’s commonly used in autonomous vehicles, game-playing, and robotics.
- Recommendation Systems: AI-driven recommendation systems analyze user behavior to suggest personalized content, products, or services. This helps improve user engagement and increases sales in e-commerce, media streaming platforms, and online marketplaces.
- Robotic Process Automation (RPA): RPA uses AI to automate repetitive and rule-based tasks, reducing the need for manual intervention. It streamlines workflows in customer support, data entry, and financial management.
- Intelligent Data Processing: By analyzing data in real-time, AI-integrated applications can automate insight extraction and make rapid decisions, which is crucial for industries like healthcare, finance, and logistics.
2. Benefits of Integrating AI into Applications
- Enhanced User Experience: AI enables applications to learn from user behavior and preferences, providing personalized recommendations, search results, and interactive experiences.
- Operational Efficiency: Automating repetitive tasks and predictive analytics can reduce the workload on employees and help them focus on strategic activities.
- Improved Decision-Making: AI analytics can process vast amounts of data in real time, providing insights for better decision-making.
- Scalability: Applications integrated with AI can adapt to evolving data sets and user needs, scaling solutions to accommodate growth.
- Competitive Advantage: Early adoption of AI technologies can give businesses a lead over competitors by offering innovative features and optimized processes.
3. Challenges of AI Integration
- Data Quality: AI relies on high-quality data for accurate predictions, and poor data quality can hamper performance.
- Ethical and Privacy Concerns: The use of personal data raises ethical and legal issues, necessitating careful data governance.
- High Costs: Developing and maintaining AI systems requires significant investment in infrastructure, talent, and R&D.
- Talent Shortage: Finding skilled AI developers and data scientists remains a challenge.
- Integration Complexity: Merging AI systems with existing IT infrastructure requires careful planning and can be technically complex.
4. Key Steps for Effective AI Integration
- Define Objectives: Clearly outline the goals and expectations of AI integration to ensure alignment with business strategies.
- Data Preparation: Gather and clean data to ensure accuracy and relevancy for training AI models.
- Choose the Right Tools: Select AI platforms and frameworks that suit the application requirements.
- Build the Model: Train and validate AI models to ensure high accuracy and performance.
- Implement and Monitor: Deploy the AI solution into the application, and continuously monitor its performance for improvements.
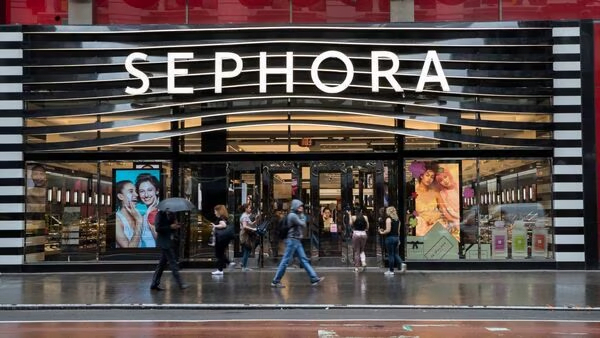
Sephora’s\ AI initiatives have led to significant improvements in customer experience and operational efficiency. The use of AI for personalized recommendations and virtual product trials has not only increased sales but also strengthened customer loyalty. Additionally, the automation of customer interactions has streamlined customer service processes, reducing wait times and improving service quality.
The success of Sephora’s AI integration illustrates the importance of aligning technology with customer needs and preferences. It also highlights the benefits of investing in innovative technologies to enhance the retail experience. However, Sephora continues to address challenges such as maintaining data privacy and managing the high costs associated with deploying cutting-edge technologies.
Sephora’s example demonstrates how AI can be effectively integrated within the retail sector to enhance the shopping experience, customize interactions, and improve operational efficiencies, setting a benchmark for others in the industry.
6. Future Trends in AI Application Development
- Users are demanding transparency in AI decision-making, leading to increased adoption of models that can explain their reasoning.
- Running AI algorithms locally on devices instead of cloud servers for real-time analysis.
There is a growing demand for AI-powered tools delivered via subscription models. - Expanding the AI capabilities of IoT devices for smarter connectivity.
- A collaborative approach to machine learning that enhances privacy by training models across decentralized devices.
Conclusion
The integration of AI into applications is a game-changer, enabling businesses to create more intelligent, efficient, and customer-centric products. Despite challenges, the potential benefits in terms of productivity, innovation, and competitiveness make AI integration a crucial strategy. By understanding the key principles and real-world examples outlined in this article, organizations can better navigate the complexities of AI application development and harness its transformative power.